3.3 Contextual semantics
The choice of tidy representation of temporal data arises from a data- and model-oriented perspective, which can accommodate all of the operations that are to be performed on the data in time-based contexts. Figure 3.1 marks where this new abstraction is placed in the tidy model, which is referred to as a “tsibble”. The “tidy data” principles are adapted in tsibble with the following rules:
- Index is a variable with inherent ordering from past to present.
- Key is a set of variables that define observational units over time.
- Each observation should be uniquely identified by index and key.
- Each observational unit should be measured at a common interval, if regularly spaced.
Figure 3.2 sketches out the data form required for a tsibble, an extension of the tidy format to the time domain. Beyond the layout, tsibble gives the contextual meaning to variables in order to construct the temporal data object, as newly introduced “index” and “key” semantics stated in definitions 1 and 2 above. Variables other than index and key are considered as measurements. Definitions 3 and 4 imply that a tsibble is tidier than tidy data, positioning itself as a model input that gives rise to more robust and reliable downstream analytics.

Figure 3.2: The architecture of the tsibble structure is built on top of the “tidy data” principles, with temporal semantics: index and key.
To materialize the abstraction of the tsibble, a subset of tuberculosis cases (World Health Organization 2018), as presented in Table 3.1, is used as an example. It contains 12 observations and 5 variables landing in a tidy data form. Each observation comprises the number of people who are diagnosed with tuberculosis for each gender at three selected countries in the years of 2011 and 2012. From tidy data to tsibble data, index and key should be declared: column year
as the index variable, and column country
together with gender
as the key variables forming the observational units. Column count
is the only measured variable in this data, but the data structure is sufficiently flexible to hold more measurements; for example, slotting the corresponding population size (if known) into the data column for normalizing the count later. Note, this data further satisfies the need for the distinct rows to be determined by index and key, and is regularly spaced over one-year intervals.
country | continent | gender | year | count |
---|---|---|---|---|
Australia | Oceania | Female | 2011 | 120 |
Australia | Oceania | Female | 2012 | 125 |
Australia | Oceania | Male | 2011 | 176 |
Australia | Oceania | Male | 2012 | 161 |
New Zealand | Oceania | Female | 2011 | 36 |
New Zealand | Oceania | Female | 2012 | 23 |
New Zealand | Oceania | Male | 2011 | 47 |
New Zealand | Oceania | Male | 2012 | 42 |
United States of America | Americas | Female | 2011 | 1170 |
United States of America | Americas | Female | 2012 | 1158 |
United States of America | Americas | Male | 2011 | 2489 |
United States of America | Americas | Male | 2012 | 2380 |
The new tsibble structure bridges the gap between raw data and the rigorous state of temporal data analysis. The proposed contextual semantics is the new add-on to tidy data in order to support more intuitive time-related manipulations and enlighten new perspectives for time series and panel model inputs. Index, key and time interval form the three pillars to this new semantically structured temporal data. Each is now described in more detail.
3.3.1 Index
Index is a variable with inherent ordering from past to present.
Time provides the contextual basis for temporal data. Time can be seen in numerous representations, from sequential numerics to the most commonly accepted date-times. Regardless of this diversity, time should be inherently ordered from past to present, so should be the index variable to a tsibble.
Index is an explicit data variable rather than a masked attribute (such as in the ts
and zoo
classes), exposing a need for more accessible and transparent time operations. It is often necessary to visualize and model seasonal effects of measurements of interest, meaning that time components, such as time of day and day of week, should be easily extracted from the index. When the index is available only as meta information, it creates an obstacle for analysts by complicating the writing of even simple queries, often requiring special purpose programming. From an analytical point of view this should be discouraged.
3.3.2 Key
Key is a set of variables that define observational units over time.
What subjects/entities are to be observed over time, leads to the second component of a tsibble–key. The key can consist of empty, single, or multiple variables identifying units measured along the way. When only a single observational unit is present in the table, no key needs to be specified. However, when multiple units exist in the data, the key should be supplied by identifying variables to sufficiently define the units. In longitudinal data, the key can be thought of as “panel” (such as in the Stata) but constrained to a single variable in existing data structures. In tsibble, the key allows for multiple variables of nesting, crossing, or union relations (Wilkinson 2005), that can be useful for forecasting reconciliation (Hyndman and Athanasopoulos 2017; Hyndman et al. 2018) and richer visualization. For example, Table 3.1 describes the number of tuberculosis cases for each gender across the countries every year. This suggests that the key comprises at least columns gender
and country
. Since country
is nested within continent
, continent
can be included in the key specification, but is not compulsory.
Each observation should be uniquely identified by index and key.
Inspired by a “primary key” (Codd 1970), a unique identifier for each observation in a relational database, the tsibble key also uniquely identifies each observational unit over time. When constructing a tsibble, any duplicates of key-index pairs will fail, because duplicates signal a data quality issue, which would likely affect subsequent analyses and hence decision making. For example, either gender
or country
alone is not enough to be the key for the tuberculosis data. Analysts are encouraged to better understand the data, or reason about the process of data cleaning when handling duplicates. Figure 3.3 reveals the tidy module with clear routes required for a tsibble. The rigidity of tsibble, as the fundamental data infrastructure, ensures the validity of subsequent temporal data analysis.
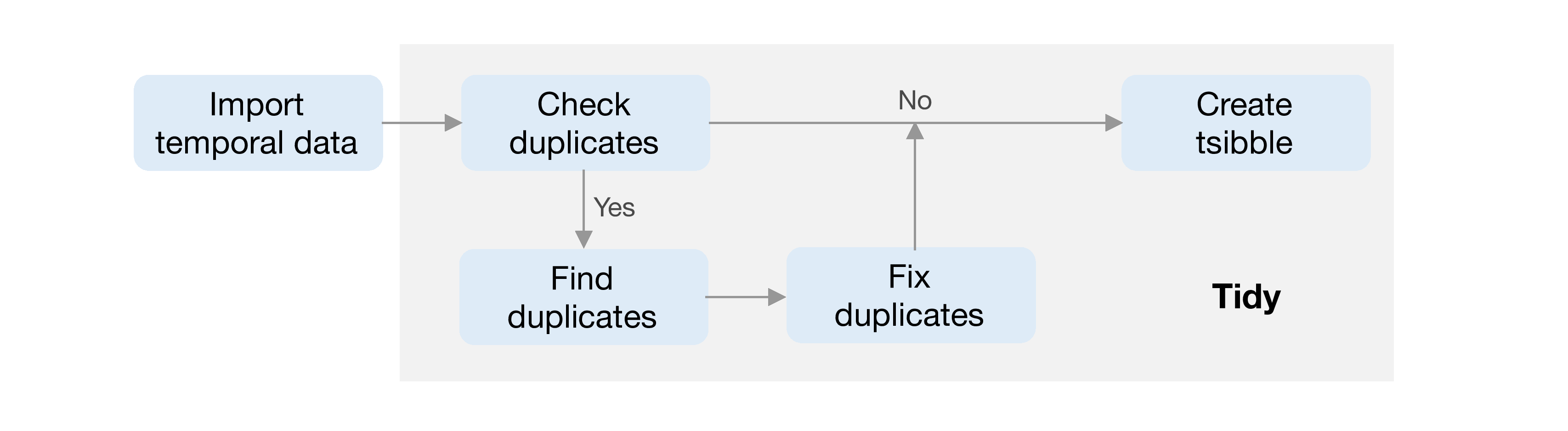
Figure 3.3: Details about the tidy stage for a tsibble. Built on top of “tidy data”, each observation should be uniquely identified by index and key, thereby no duplicated key-index pairs.
Since observational units are embedded, modeling and forecasting across units and time in a tsibble will be simplified. The tsibble key plays the role of the central transit hub in connecting multiple tables managed by the data, models, and forecasts. This neatly decouples the expensive data copying from downstream summarization, which can significantly reduce the required storage space.
3.3.3 Interval
Each observational unit should be measured at a common interval, if regularly spaced.
The principal divide of temporal data is regularly versus irregularly spaced data. Event data typically involves irregular time intervals, such as flight schedules or customer transactions. This type of data can flow into event-based data modeling, but would need to be processed, or regularized, to fit models that expect data with a fixed-time interval.
There are three possible interval types: fixed, unknown, and irregular. To determine the interval for regularly spaced data, tsibble computes the greatest common divisor as a fixed interval. If only one observation is available for each unit, which may occur after aggregating data, the interval is reported as unknown. When the data arrives with irregular time, like event data, the interval would be specified as irregular, to prevent the tsibble creator attempting to guess an interval.
To abide by the “tidy data” rules – “Each type of observational unit should form a table” – in a tsibble each observational unit shares a common interval. This means that a tsibble will report one single interval, whether the data has a fixed or mixed set of intervals. To handle mixed interval data, it should be organized into separate tsibbles for a well-tailored analysis.
This tiny piece of information, the interval, is carried over for tsibble-centric operations. For example, this makes implicit missing time handling convenient, and harmoniously operates with statistical calculations, and models, on seasonal periods.