4.2 Categories of temporal missing data
Missing values in time can occur in many different patterns. Figure 4.1 presents a classical time series, monthly totals of international airline passengers from 1949 to 1960 (Box and Jenkins 1990), with simulated gaps of missing values arising from four different patterns:
- sporadically, where data is missing at random time points, which will be called Missing at Occasions (MO).
- periodically, for example, missing every Tuesday, which will be called Missing at Periodic time (MP). This could be thought of as structural missing values.
- functionally, such as more frequent with time, as might happen in a longitudinal study where participants drop out increasingly as time progresses. This will be called Missing at Functional (MF).
- in runs, for example, in an instrument breakdown, it might take some time period to repair the machine. This will be called Missing at Runs (MR).
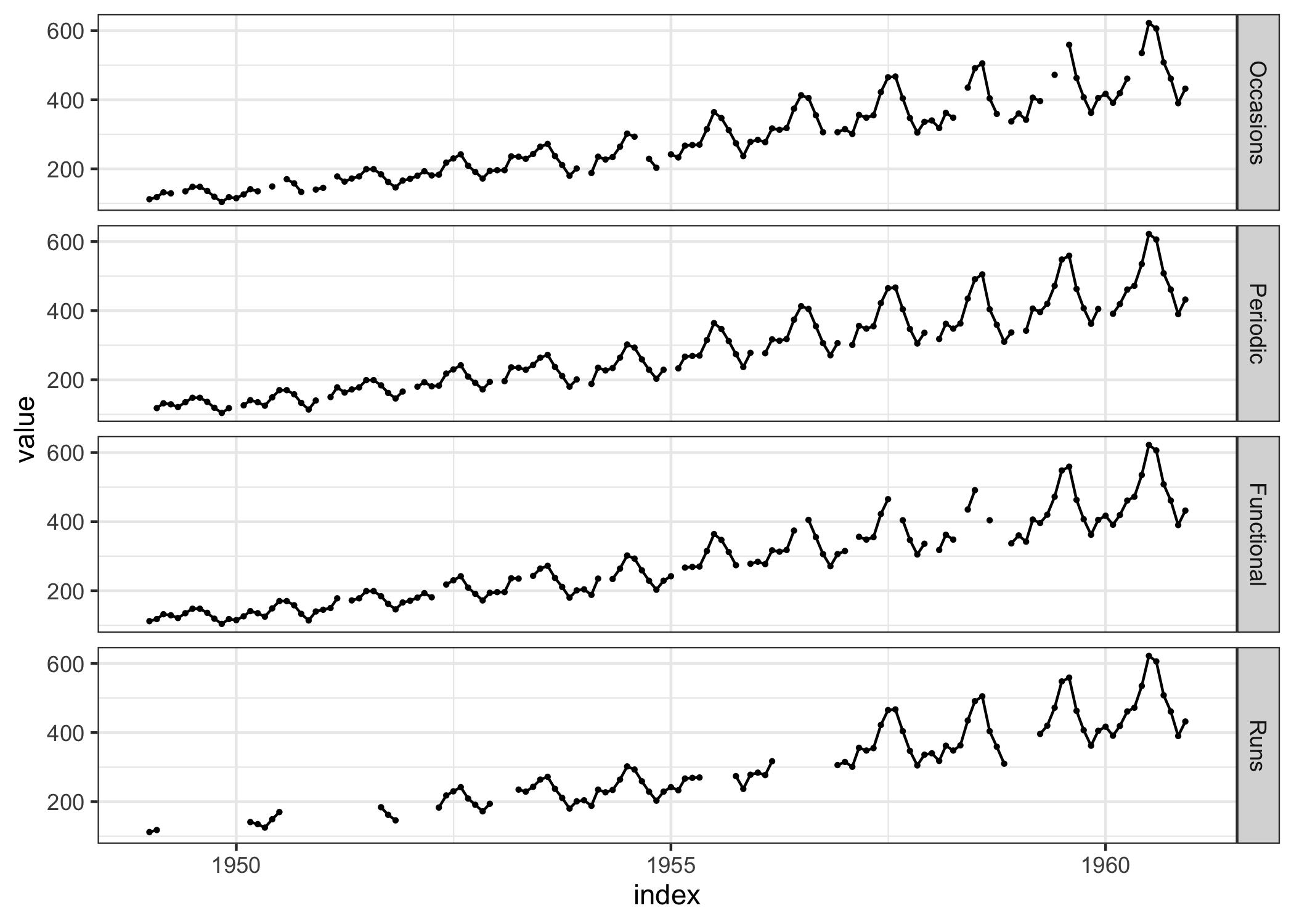
Figure 4.1: Line plots of a series spiked with four different types of temporal missing patterns. It is hard to discern the difference in the patterns from line plots, which motivates development of new graphical methods for exploring temporal missingness.
This categorization may not be exhaustive, although with combinations these four types can form a wide range of temporal missing data patterns.
Some of these types can be mapped to probability nomenclature for missing values. MO mirrors MCAR, where missings are completely at random. MP and MF are forms of MAR, where a known variable could be used to build imputation models. MR does not have an analogy.
It is difficult to detect the missing patterns, or discern the difference, from Figure 4.1. This is a typical way to plot time series in the presence of missing values, but it is not a good diagnostic plot. Thus, the motivation for this new work, a desire to provide better diagnostic plots for exploring temporal missings, that neatly integrates with the tidy data workflow. To lubricate this work, a new data structure is developed, and discussed in the next section.